Climate change is one of the most critical challenges faced by humans and our planet. Researchers at ISSE, working closely with the Climate Change Science Institute at Oak Ridge National Lab, are advancing our understanding of climate change and its impacts on human and natural systems. We use Earth system modeling, integrated ground and remote sensing observations, and advanced data analytical tools to study climate change and its effects on water availability, soil moisture, wildfires, and vegetation. Team members are Drs. Yaoping Yao, Yulong Zhang, Jiafu Mao, Joshua Fu, Mingzhou Jin.
Jump to:
Water Availability
Water (or runoff) is an essential resource for the terrestrial biosphere as well as for human society; thus, it is important to detect and understand the potential drivers of changes in the hydrological cycle. Here We studied annual and seasonal runoff in the contiguous United States and streamflow in the Columbia River Basin for the period 1950 – 2010 and 1950 – 2008, respectively. For forcings, the effects of climate change and variability, CO2concentration, nitrogen deposition, and land use and land cover change are used in both studies. Monthly observations of runoff from WaterWatch of the United States Geological Survey, and an ensemble of semi-factorial land surface model simulations were used to quantify the effects due to external forcings. United States runoff had significant and insignificant increases in the east, north, and south, and a strong significant decrease in the west. These changes were detected in the effects of climate change and variability, but could not be attributed due to the dry bias in the precipitation driver leading to underestimation in the model simulations. However, for the Columbia River Basin, the changes in annual total, center of timing of, and summer mean streamflow were attributed to climate change and variability. The most significant changes were the declines in the June – October months. On average, these months account for approximately 49% of the annual total flow. More specifically, the greatest decline was 28% for June which comprised approximately 22% of the total annual flow.
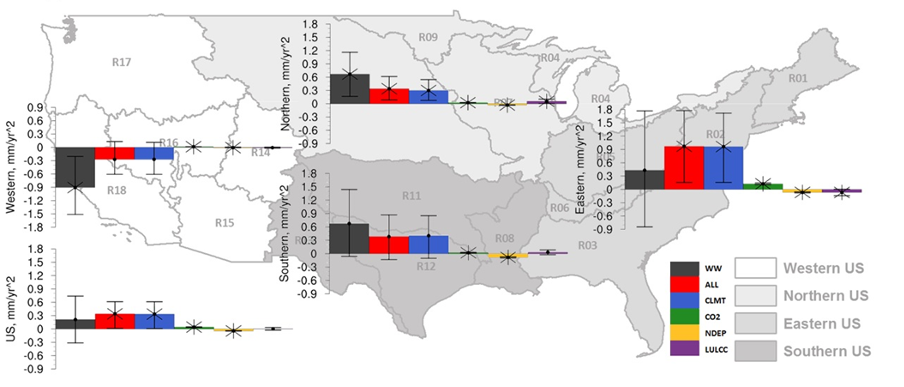
Sample Publications
Forbes, W.L., Mao, J., Ricciuto, D.M., Kao, S.C., Shi, X., Tavakoly, A.A., Jin, M., Guo, W., Zhao, T., Wang, Y. and Thornton, P.E., 2019. Streamflow in the Columbia River Basin: Quantifying changes over the period 1951‐2008 and determining the drivers of those changes. Water Resources Research, 55(8), pp.6640-6652.
Forbes, W.L., Mao, J., Jin, M., Kao, S.C., Fu, W., Shi, X., Riccuito, D.M., Thornton, P.E., Ribes, A., Wang, Y. and Piao, S., 2018. Contribution of environmental forcings to US runoff changes for the period 1950–2010. Environmental Research Letters, 13(5), p.054023.
Wang, Y., Sivandran, G. and Bielicki, J.M., 2018. The stationarity of two statistical downscaling methods for precipitation under different choices of cross‐validation periods. International Journal of Climatology, 38, pp.e330-e348.
Soil Moisture
Soil moisture datasets are critical to understanding the global water, energy, and biogeochemical cycles and benefit extensive societal applications. We developed seven global, gap-free, long-term (1970–2016), multi-layer (0–10, 10–30, 30–50, and 50–100 cm) soil moisture datasets at monthly 0.5° resolution (available at https://doi.org/10.6084/m9.figshare.13661312.v1) by synthesizing a wide range of soil moisture datasets using three statistical methods (unweighted averaging, optimal linear combination, and emergent constraint). The merged datasets outperformed their source datasets when evaluated against in situ observations and the latest gridded datasets that did not enter merging because of insufficient spatial, temporal, or soil layer coverage. Assessed against in situ observations, the global mean bias of the synthesized soil moisture data ranged from −0.044 to 0.033 m3/m3, root mean squared error from 0.076 to 0.104 m3/m3, and Pearson correlation from 0.35 to 0.67. The merged datasets also showed the ability to capture historical large-scale drought events and physically plausible global sensitivities to observed meteorological factors. Three of the merged products that were based on satellite, reanalysis, and offline land surface model source datasets were finally recommended for future applications because of their better performances than the Earth system model–dependent merged datasets. These merged datasets add value over existing soil moisture datasets because of the performance improvement and harmonized spatial, temporal, and vertical coverages, and they provide a new foundation for scientific investigation and resource management.
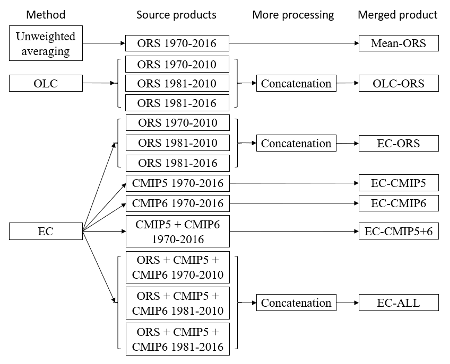
Sample Publications
Wang, Y., Mao, J., Jin, M., Hoffman, F.M., Shi, X., Wullschleger, S.D. and Dai, Y., 2021. Development of Observation-based Global Multi-layer Soil Moisture Products for 1970 to 2016. Earth System Science Data, Accepted.
Wildfires
Understanding historical wildfire variations and their environmental driving mechanisms is key to predicting and mitigating wildfires. Using recent satellite-derived wildfire products and simulations from version v1.0 of the land component of the US Department of Energy’s Energy Exascale Earth System Model (E3SM land model [ELM] v1) driven by three different climate forcings, we investigated the interannual variability (IAV) and its climatic sensitivity of the burned area globally and across nine biomes from 1997 to 2018. We found that (1) the ELM simulations generally agreed with the satellite observations in terms of IAV magnitudes, regional contributions, and covariations with climate factors of the burned area, confirming the robustness of the ELM to the usage of different climate forcing sources; (2) tropical savannas, tropical forests, and semi-arid grasslands were primary contributors to the global burned area IAV, collectively accounting for 71.7%–99.7% of the global wildfire IAV estimated by both the satellite observations and ELM simulations; (3) precipitation was a major fire suppressing factor and dominated the IAVs of global and regional burned area, and temperature and shortwave solar radiation were mostly positively related with burned area IAVs; and (4) noticeable local discrepancies between the ELM and remote-sensing results occurred in semi-arid grasslands, croplands, boreal forests, and wetlands, likely caused by uncertainties in the current ELM fire scheme and the imperfectly derived satellite observations. Our findings reveal the spatiotemporal diversity of wildfire variations, regional contributions, and climatic responses, and can provide new insights for wildfire modeling, prediction, and management.
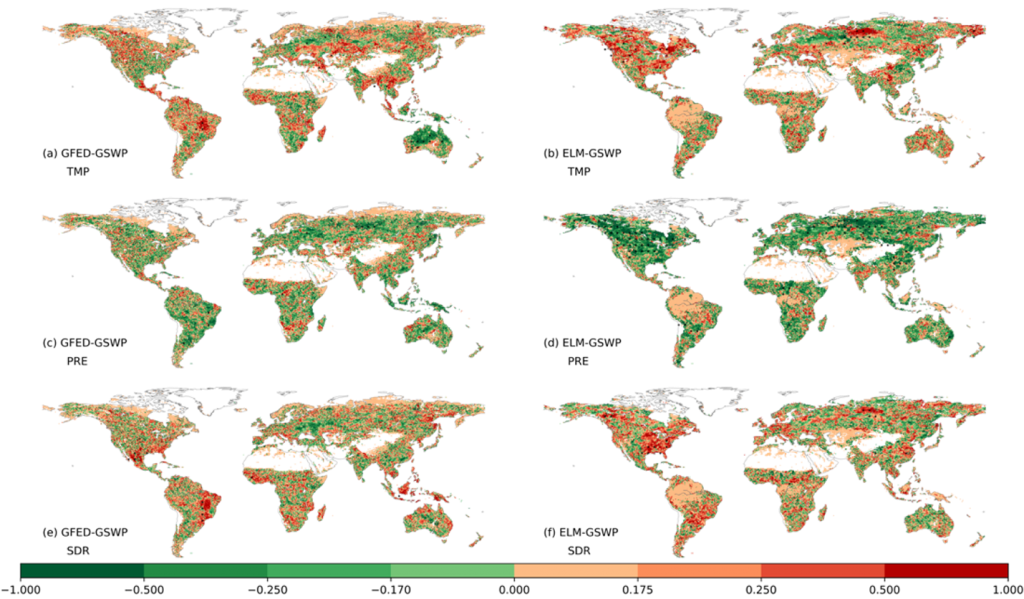
Sample Publications
Tang, R., Mao, Jiafu, Jin. M., Chen, A., Yu, Y., Shi, X., Zhang, Y., Hoffman, F.M., Xu, M. and Wang, Y., 2021. Interannual variability and climatic sensitivity of global wildfire activity. Advances in Climate Change Research.
Chen, A., Tang, R., Mao, J., Yue, C., Li, X., Gao, M., Shi, X., Jin, M., Ricciuto, D., Rabin, S. and Ciais, P., 2020. Spatiotemporal dynamics of ecosystem fires and biomass burning-induced carbon emissions in China over the past two decades. Geography and Sustainability, 1(1), pp.47-58.
Vegetation
The terrestrial biosphere on Earth is breathing continuously by inhaling carbon dioxide from the atmosphere (a process known as “photosynthesis”) and exhaling water vapor into the atmosphere (a process known as “evapotranspiration”). These ‘invisible’ gas exchanges are key drivers of global carbon and water cycles, serving important roles in regulating climate system. We have worked on modeling and understanding these dynamic processes at the global scale based on remote sensing, ecological modeling, and ground observations. Specific research are related to eco-hydrological model development, Earth’s greening, El Niño-Southern Oscillation, and climate drying and land use/cover change.
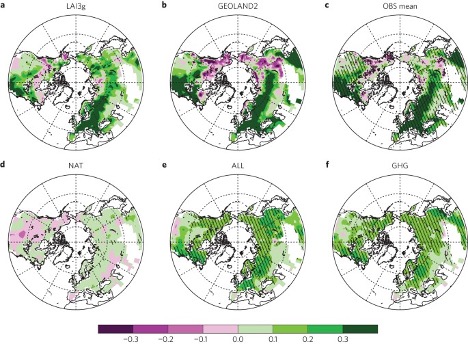
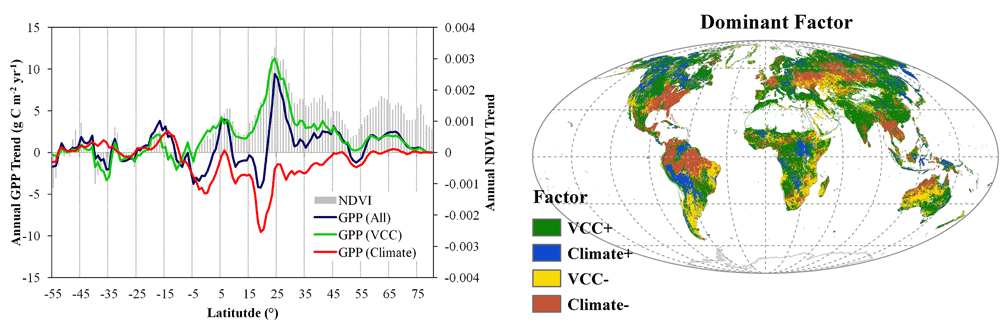
Sample Publications
Y. Zhang, C. Song, T. Hwang, K. Novick, J. W. Coulston, J. Vose, M. P. Dannenberg, C. R. Hakkenberg, J. Mao, C. E. Woodcock, 2021. Land cover change-induced decline in terrestrial gross primary production over the conterminous United States from 2001 to 2016. Agricultural and Forest Meteorology [Accepted]
Y. Zhang, C. Song, L. E. Band, G. Sun, J. Li, 2019. No proportional increase of terrestrial gross carbon sequestration from the greening Earth. Journal of Geophysical Research: Biogeosciences https://doi.org/10.1029/2018JG004917
Y. Zhang, M.P. Dannenberg, T. Hwang, C. Song, 2019. El Niño-Southern Oscillation-induced variability of terrestrial gross primary production during the satellite era. Journal of Geophysical Research: Biogeosciences https://doi.org/10.1029/2019JG005117
Y. Zhang, C. Song, L. E. Band, G. Sun, J. Li, 2017. Reanalysis of global terrestrial vegetation trends from MODIS products: browning or greening? Remote Sensing of Environment. https://doi.org/10.1016/j.rse.2016.12.018
J. Mao, A. Ribes, B. Yan, X. Shi, R. P. Thornton, R. Séférian, P. Ciais, R. B. Myneni,, H. Douville, S. Piao, Z. Zhu, R. E. Dickson, Y. Dai, D. M. Ricciuto, M. Jin, F. M. Hoffman, B. Wang, M. Huang, and X. Lian,, 2016. Human-induced greening of the northern extratropical land surface. Nature Climate Change, 6(10), pp.959-963.
Y. Zhang, C. Song, G. Sun, L. E. Band, S. McNulty, A. Noormets, Q. Zhang, Z. Zhang, 2016. Development of a coupled carbon and water model for estimating global gross primary productivity and evapotranspiration based on eddy flux and remote sensing data. Agricultural and Forest Meteorology. https://doi.org/10.1016/j.agrformet.2016.04.003
Y. Zhang, C. Song, G. Sun, L. E. Band, A. Noormets, Q. Zhang, 2015. Understanding moisture stress on light-use efficiency across terrestrial ecosystems based on global flux and remote sensing data. Journal of Geophysical Research: Biogeosciences. https://doi.org/10.1002/2015JG003023